Cummings Employs Machine Learning to Tackle Addiction Crisis
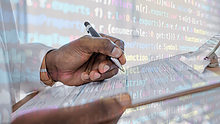
Baltimore is among the epicenters of the nationwide substance abuse crisis. The city was recently named the “Overdose capital of the U.S” after a joint investigation by The New York Times and Baltimore Banner found it averaged 1,000 overdose deaths per year, a death rate nearly double of any other large American city.
In a concerted effort to curtail overdose deaths and improve treatment for people struggling with substance abuse, researchers at the University of Maryland (UMD) and University of Maryland, Baltimore (UMB) have partnered with a regional drug treatment center to apply machine-learning techniques to electronic medical record (EMR) data.
By using EMR to analyze data from patients including race, gender, location, traumas suffered, educational background and income, the cross-institutional team is hoping to identify trends that allow treatment to be better tailored to the individual and improve recovery rates.
“Our complex histories, individual abilities and family situations are all very personal to us, but there's going to be some commonalities among people suffering from addiction,” says Michael Cummings, a professor of biology with an appointment in the University of Maryland Institute for Advanced Computer Studies (UMIACS) who is one of the researchers on the project.
Cummings’ partners on the project include Edward Bernat, an associate professor of psychology at UMD, and Fadia Shaya, a Distinguished University Professor in the school of pharmacy at UMB. Yi Chen, a fifth-year doctoral student in UMD’s biological sciences program advised by Cummings, is also involved with the project.
Their work is supported by a $50,000 grant from the Institute for Clinical and Translational Research and the University of Maryland, Baltimore.
Bernat has extensively researched substance use disorders and its intersection with psychopathology. For the past few years, he has been working directly with Tuerk House— an inpatient drug center and crisis stabilization center in West Baltimore that sees 500–600 patients a month. Bernat provides ongoing clinical intake diagnostic services for the center’s clinical team.
Not long after he began conducting evaluations of the EMRs for patients arriving at Tuerk House, Bernat saw a need to involve machine learning to get the most out of the data he was viewing. This was due to the larger amount of data, from a larger number of patients.
“That’s where machine learning often has a big advantage—going through all of those different variables, and getting it crunched down into what’s actually meaningful with the minimal data available,” says Bernat.
The researchers plan to run machine learning algorithms on up to 10,000 of Tuerk House’s unique intake records from 2022 and 2023, with a focus on additional hurdles that Black substance users face that can impact their path to recovery.
African Americans complete substance abuse treatment only 40% of the time, which is 10% less than their White counterparts, according to a 2016 study published in the Journal of Substance Abuse Treatment. The study said that factors like socioeconomic status— specifically high school attainment and employment—are both associated with higher completion rates.
“If we find some subpopulations of people with certain characteristics are more at risk for relapse or something like that, then we can modify treatment for those sets of individuals.,” Cummings says.
Tuerk House’s partnership with UMD researchers for this project is part of a broader developing collaborative relationship to advance health disparities and treatment research relevant to Black substance users.
The inpatient drug center is in a majority black city that has been disproportionately damaged by the addiction epidemic, one that Chen is incredibly familiar with.
Chen moved to Baltimore to attend Johns Hopkins University, where he completed his B.A. in biology in 2014. For four years he did community service at Stepping Stone Ministry, a faith-based organization on the Hopkins campus that gave aid to the local homeless community, only stopping in 2020, when COVID–19 forced him to do so.
“Substance use disorder and homelessness are highly comorbid,” Chen says. “So, in some ways, this project is another way of serving the same population from that part of my life using a different set of skills.”
This project contrasts Chen’s previous work which has centered around molecular biological data sets, posing occasional challenges in refining and understanding data.
Chen noted the significance of the collaboration of experts from across various fields for this project.
“Substance use disorder is a complex disorder,” he says. “This project can hopefully address that by virtue of being a collaborative work involving psychopathology, social determinants, and machine learning.”
Looking ahead, Bernat envisions a dashboard wherein a patient completes the intake assessment at Tuerk House, and the system the research team is building will suggest individualized treatment plans based on the data received.
“Our local goal with is to develop something with [Tuerk House] clinicians that they can use quickly and efficiently,” he says.
Bernat and Cummings both emphasized that the overall goal is to understand different populations and improve the success rate of addiction treatment through a data-driven, personalized approach.
“That’s the ultimate benchmark we’re looking to achieve,” says Cummings. “Hopefully, data science and machine learning can provide some insights on how specialized health care can be improved and become more individualistic.”
— Story By Shaun Chornobroff, UMIACS communications group